Introduction
The Bardavon Index was created by a team of data scientists at Bardavon. It is a collection of statistical and predictive analytic models used to optimize the care for each individual patient. The goal of the Bardavon Index is to provide actionable information about a physical or occupational therapy case to various stakeholders to ensure the best care possible is provided to the patient.
The Bardavon Index consists of four components (see below). These will be described in detail in the following section.
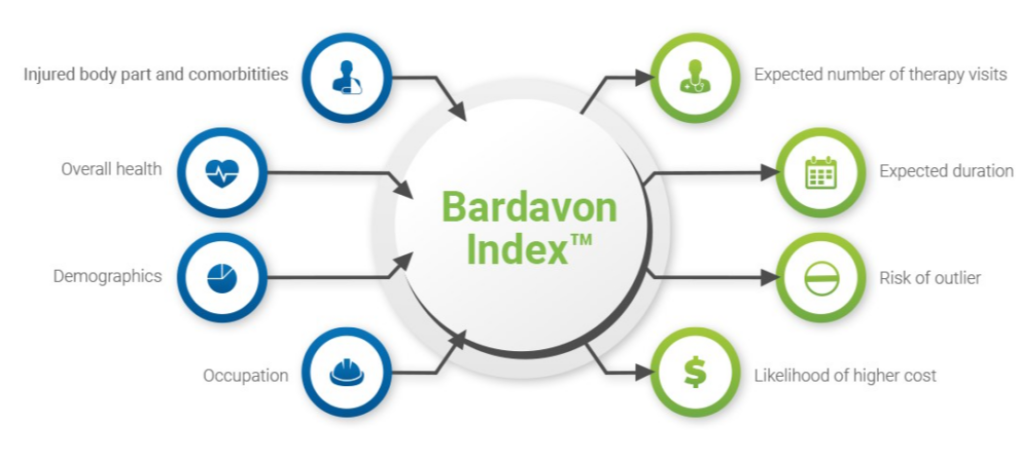
- Diagnostic Average. Averages and outlier thresholds for the number of visits and duration based on the medical diagnosis and surgical status of a case
- Outlier Risk Indicator. Score indicating the risk of a specific case becoming an outlier (See definition of an outlier in the section below)
- Personalized Utilization Prediction. Prediction of the number of visits and duration for a specific case that factors in the complexity of the case beyond the diagnosis
- Provider Quality Index. Objective measurement of the quality of a therapy provider, clinic location, and physical therapy (PT) company
Details of The Bardavon Index Components
Diagnostic Average
The diagnostic average defines the average number of therapy visits needed to return the patient back to their initial musculoskeletal abilities prior to the injury. It also defines the average elapsed time (calendar days) this treatment will take. Included in the diagnostic average are the outlier threshold values for visits and duration.
The outlier threshold is defined as the number of visits and duration at which 85% of the cases in Bardavon’s historical data set have ended at or below. The diagnostic average is based on the medical diagnosis (ICD10) and surgical status (the treatment occurring following surgery).
A few sample rows from the Diagnostic Average Table are shown below:
ICD Code | Surgical Status | Avg # Visits | # Visits Outlier Threshold | Avg Duration | Duration Outlier Threshold |
---|---|---|---|---|---|
M23.8X1 | Nonsurgical | 10 | 17 | 35 | 65 |
M23.8X1 | Surgical | 17 | 28 | 62 | 107 |
M19.011 | Nonsurgical | 11 | 16 | 41 | 66 |
M19.011 | Surgical | 26 | 43 | 87 | 146 |
Outlier Risk Indicator
The Outlier Risk Indicator is based on a predictive model that scores each case to identify the level of risk of it becoming an outlier. This model leverages information collected by Bardavon’s proprietary clinical guidance software, bNOTES®, as well as other sources. The data includes the patient’s medical history, injury type, job demands, and other confounding factors to calculate the risk.
There are two Outlier Risk Indicators for every case: the initial outlier risk and the current outlier risk. The initial Outlier Risk Indicator is based only on data available after the initial evaluation. This risk indicator stays static from that point forward. The current Outlier Risk Indicator updates throughout the course of care. This model considers current case progression (visits utilized and case duration). It also considers patient behavior, including cancellations and no-shows for appointments.
These risk indicators are shared with key stakeholders for clinical best practices and therapy management. They are also used to inform the Bardavon Clinical Quality Care Management Team on needed oversight for the case and are used to prioritize the cases they review.
Personalized Utilization Prediction
The personalized utilization prediction is used to predict the number of visits and case duration that will be used for each case. Like the Outlier Risk Indicator, this is based on a predictive model that leverages the data collected by bNOTES and other sources.
Like the Outlier Risk Indicator, this prediction is based on the combination of the medical diagnosis and each injured worker’s case complexity. While the Outlier Risk Indicator focuses on factors that will cause utilization to be significantly above the diagnostic average, this model also looks at factors that will cause the utilization to be below the diagnostic average.
This is calculated after the initial evaluation and remains static after that point. This prediction is input into the provider quality index to determine how well a provider performed on each case (how the actual utilization compares to the personalized utilization prediction).
Provider Quality Index
Bardavon network therapy providers are scored on the quality of functional outcomes they achieve, as well as how well they document each case and the treatments they perform for each visit. The outcome scores are measured by comparing the results for each case to the expected visits and duration predicted by the Personalized Utilization Prediction Model for that specific case. This model accounts for individual case complexity. The Provider Quality Index provides an objective measurement of the quality of each therapy provider, clinic location, and PT company within the Bardavon Network, with enough closed cases to enable a valid measurement.
The scores are based on:
- Quality functional outcomes
- Documentation completeness and timeliness
- Type and number of treatments performed
- Time from referral placed to initial evaluation (only used for clinic location and PT company scores, not therapist scores)
The Provider Quality Index is used to drive:
- Prioritization of case reviews
- Coaching during quality reviews
- Referral placement
Methodology
The Bardavon Index is used to provide near real-time information about physical and occupational therapy cases, enabling therapy providers, claims examiners, risk managers, and nurse case managers to proactively manage their open workers’ compensation cases. It is also used by the Bardavon Clinical Quality Care Management team when performing clinical case reviews and is leveraged by the automated digital case reviews that are performed nightly.
The Bardavon Index is based on the analysis performed on a very large and ever-growing number of completed physical therapy and occupational therapy cases performed in the Bardavon Network. The index is based primarily on the rich clinical treatment data collected within the bNOTES system. But it also includes the billing data for each visit and information provided when the referral is received. For many referrals, this includes detailed job descriptions or job assessments.
The diagnostic average values are calculated based purely on the medical diagnosis (ICD10) and the surgical status of the case. The average number of visits and duration for each completed case is calculated. We also calculate the variation of each of these values for each diagnosis in order to determine the outlier threshold. Single-visit cases are excluded from the calculations. However, outlier cases are included. The Bardavon Data Science team utilizes machine learning, specifically regression analysis, to create the predictive models that comprise the other three components of The Bardavon Index: Outlier Risk Indicator, Personalized Utilization Prediction, and Provider Quality Index.
Three of the four components of the Bardavon Index leverage data specific to the individual patient. However, one (by design) only includes the diagnosis and surgical status in the calculation. Cases with the same diagnosis and surgical status will be compared against the same diagnostic averages. But, the other components of the index will vary based on the specifics of the patient and case. See the table below:
Bardavon Index Component | Diagnosis / Surgical Status | Patient/Case/Job Specific Attributes |
---|---|---|
Diagnostic Average | ✔ | |
Outlier Risk Indicator | ✔ | ✔ |
Personalized Utilization Prediction | ✔ | ✔ |
Provider Quality Index | ✔ | ✔ |
Outliers
Much of the Bardavon Index is based on the identification and prevention of outliers. So, it is important to understand how Bardavon defines an outlier. We define an outlier as a case with more visits or longer duration than approximately 85% of our cases with the same diagnosis and surgical status. For each diagnosis, we calculate the average (mean) number of visits and the Standard Deviation of the number of visits. Leveraging the empirical rule, we calculate the outlier threshold as equal to one standard deviation above the mean. We use the same calculations for determining the outlier threshold for case duration.
- Number (#) Visits Outlier Threshold = Mean (# Visits) + Standard Deviation (# Visits)
- Duration Outlier Threshold = Mean (Duration) + Standard Deviation (Duration)
Note: In some scenarios, people will classify an outlier as Mean + 2 Standard Deviations. We want to use this lower threshold to manage the cases in our network more precisely.
Data-Informed Decisions
The Bardavon Index is used to enable data–informed decisions along the progression of care. Below is an example of how key values from the index are communicated to stakeholders in a simple graphic showing where the current case sits relative to these values.
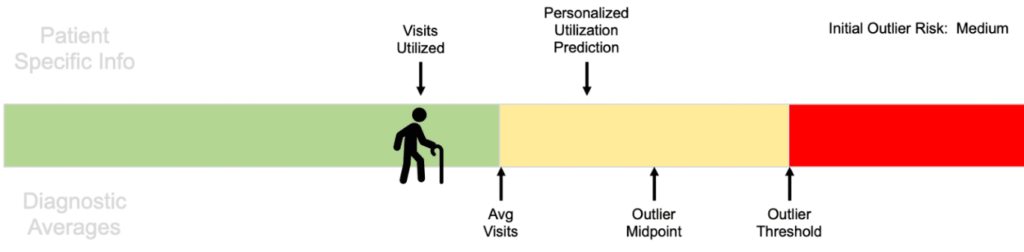
Impact of bNOTES Usage on the Bardavon Index
The vast majority of our cases are documented in bNOTES. However, about 15% of our cases are documented elsewhere. For these cases, we do not have the rich clinical data that bNOTES provides. However, we are still able to leverage the Bardavon Index. The table below shows the impact of bNOTES usage on each component.
Bardavon Index Component | Impact of bNOTES Usage |
---|---|
Diagnostic Average | None |
Outlier Risk IndicatorPersonalized Utilization Prediction | Calculated for all cases. Prediction is not as accurate for non-bNOTES cases as it is for bNOTES cases since some of the data used to make the prediction is not available. |
Provider Quality Index | Calculated for all providers. Timeliness and completeness of documentation are not considered for non-bNOTES cases. The rating is not as accurate for non-bNOTES providers since some of the data used to score performance is not available. |
Comparison of the Diagnostic Averages with ODG
The diagnostic average component of the Bardavon Index is very similar to ODG. Bardavon is a customer of ODG and leverages the ODG values as well as the Bardavon Index values in our management of the Bardavon Network. The primary difference is that the diagnostic averages also include the outlier threshold values.
The diagnostic average values are typically very close to the ODG values for the same diagnosis and surgical status:
- Bardavon Diagnostic Average and ODG are equal for 19% of our cases
- Bardavon Diagnostic Average and ODG are within one visit for 53% of our cases
- Bardavon Diagnostic Average and ODG are within two visits for 71% of our cases
As shown in the charts below, the Bardavon Diagnostic Average is more granular/precise than the ODG values.
Distributions for all cases:
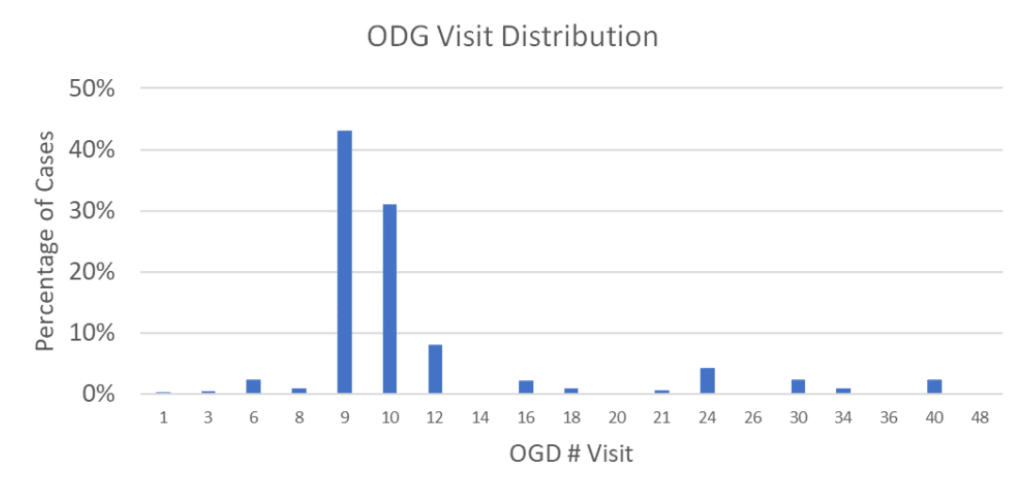
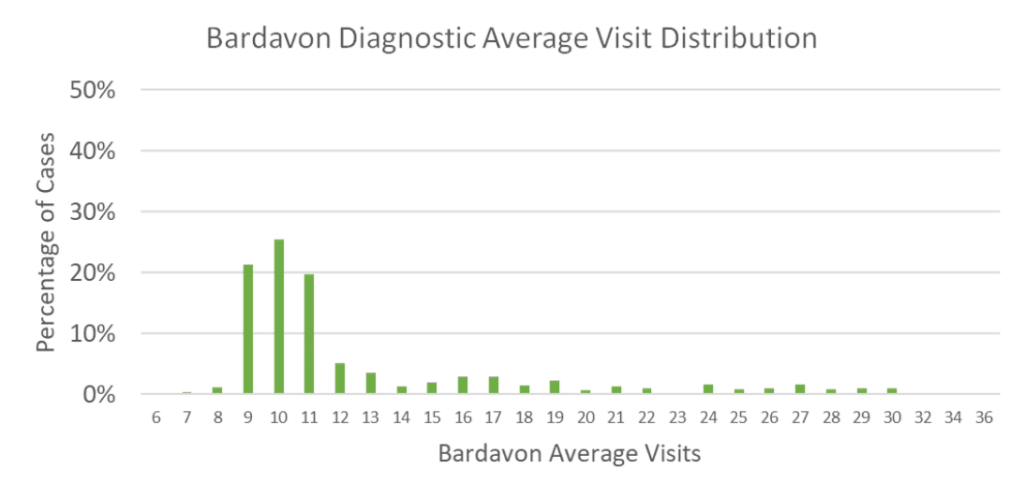
Distributions for Nonsurgical Cases:
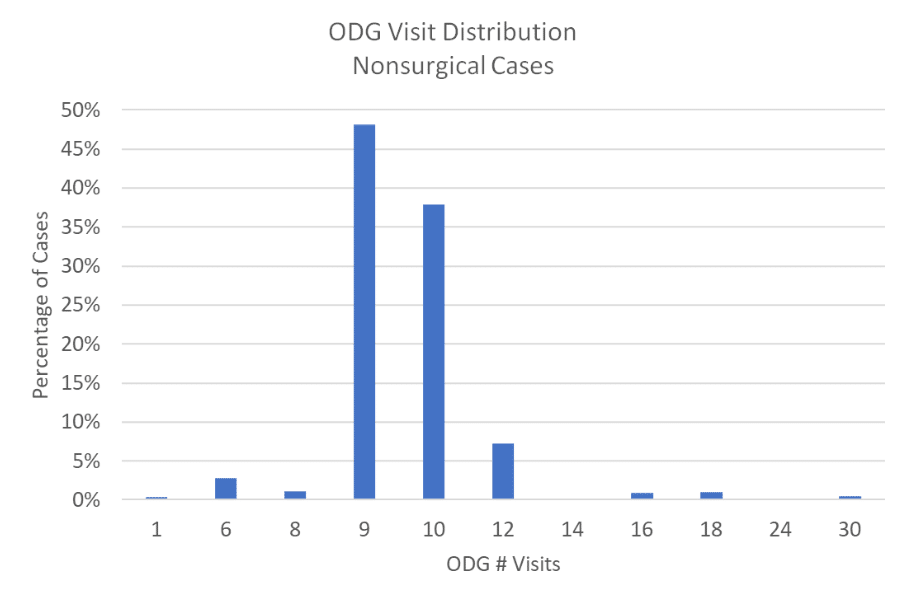
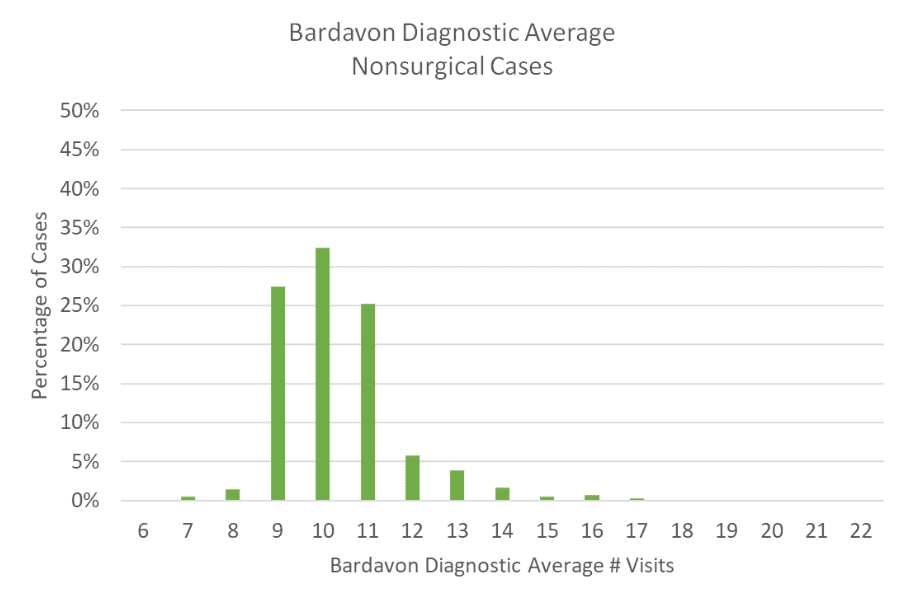
Distributions for Surgical Cases:
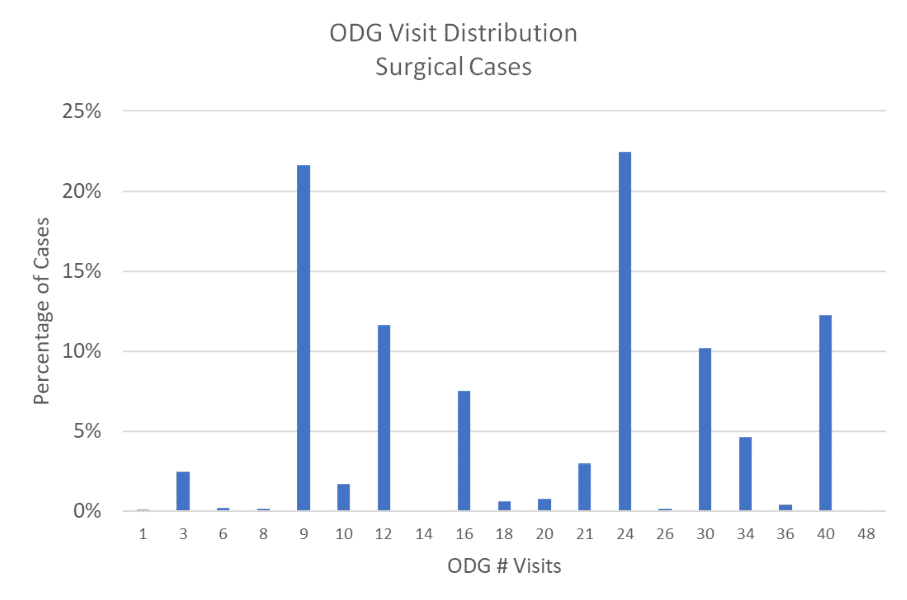
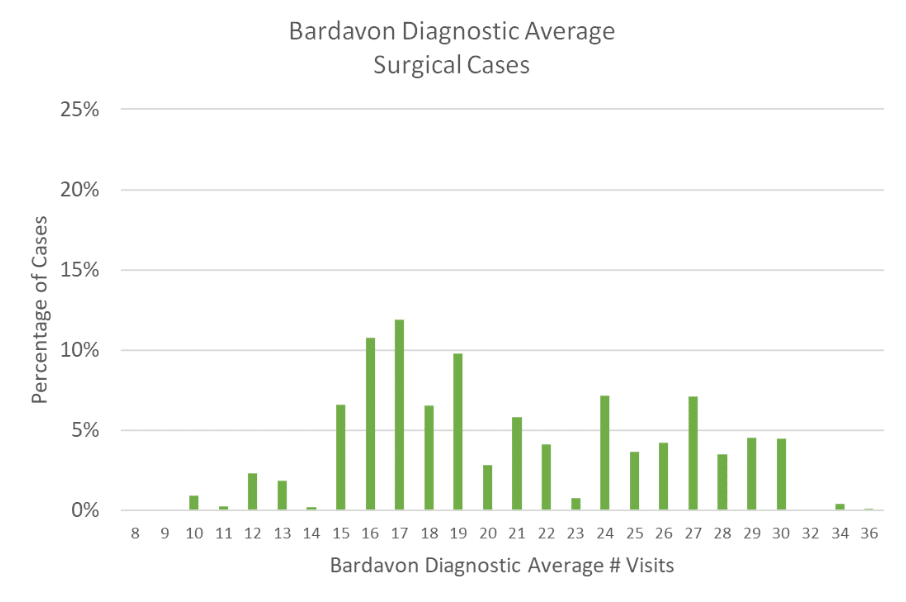
Conclusion
The Bardavon Index is a valuable tool for managing physical and occupational therapy cases. It was built by a team of data scientists at Bardavon, primarily leveraging data from bNOTES. The index leverages detailed clinical, job, script, referral, and billing data from a large and ever-growing number of completed therapy cases to identify averages and variations in therapy visits and duration. They have also created and trained predictive models on this data, which enables us to identify high-risk cases early in the continuum of care and to provide this risk information to various stakeholders that can leverage this information to help get the best possible outcome for the patient.
Key Takeaways
- The Diagnostic Averages component of the Bardavon Index provides very granular and precise values for the averages for each diagnosis and surgical status. It also provides outlier thresholds based on the variability of utilization for each diagnosis.
- The Outlier Risk Indicator and Personalized Utilization Prediction components of The Bardavon Index leverage machine learning and data science to provide early predictions of the risk associated with each case and the expected utilization for each case.
- The Provider Quality Index component of the Bardavon Index provides an objective measurement of the quality of the therapy providers, clinic locations, and PT companies within the Bardavon Network. This is the first such measurement in the industry.